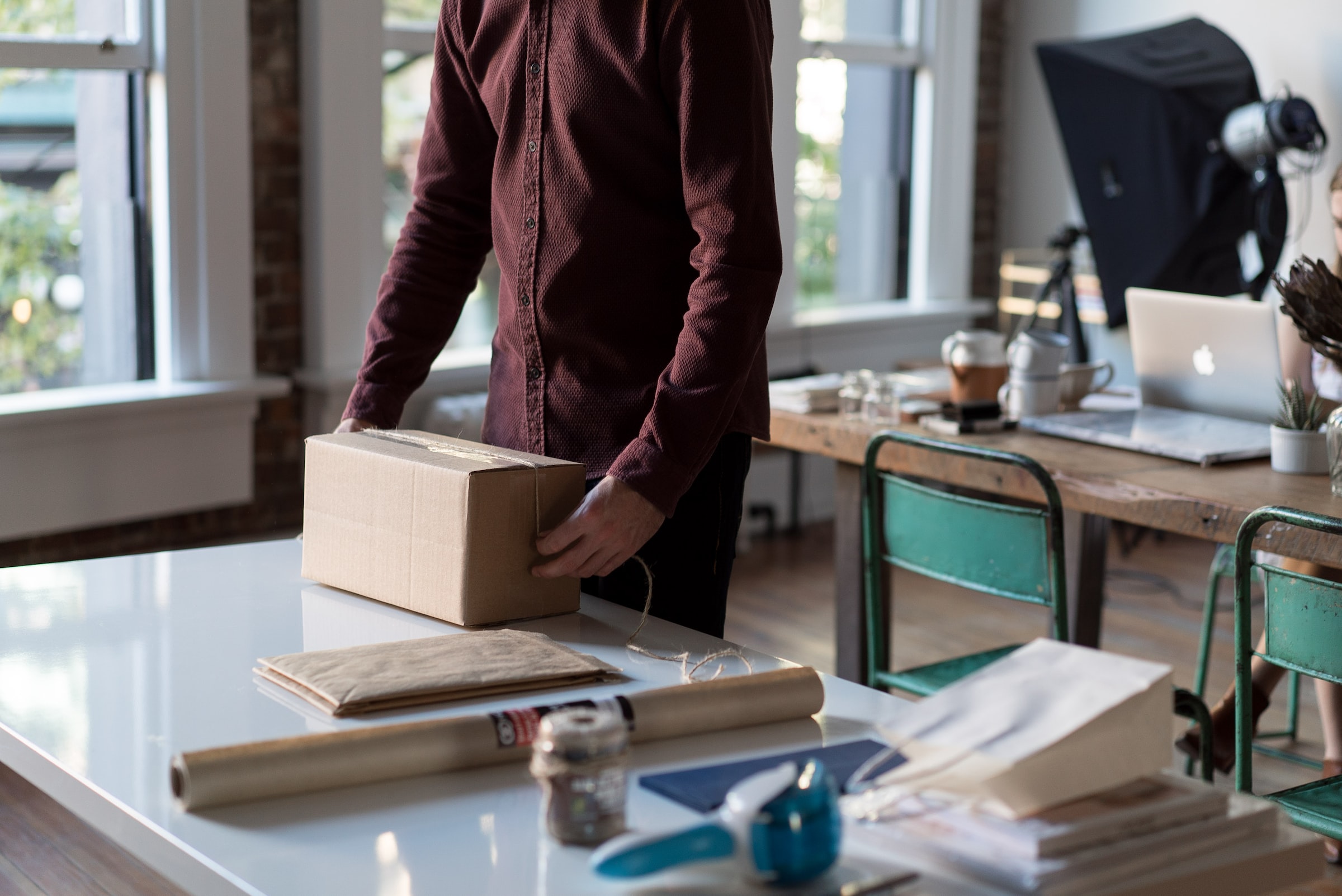
Amid global economic turmoil, using AI to improve online experiences and extract the most value from every investment is more important than ever.
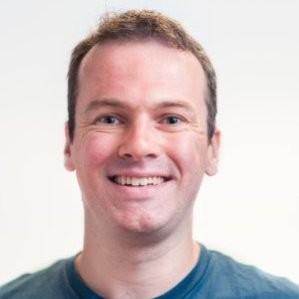
The advertising industry is a pioneer of AI and machine learning; harnessing the technologies to deliver personalised experiences that ensure the right content is put in front of the right people at the right time.
AI News caught up with Tim van Kasteren, Head of Engineering at Adevinta, to learn more about how one of Europe’s online classifieds leaders is using AI.
AI News: From the top, how is AI improving online classifieds?
Tim van Kasteren: Online classifieds are a form of two-sided marketplaces where a buyer and a seller come together to close a deal. Historically, we are a group of marketplaces where we connect people who transact outside of our platform. We are now adding more and more services to allow people to transact safely on our platforms (integrated shipping options, payments through the platform, buyer protection, etc).
We apply AI in many stages of the user journeys of both the buyer and seller.
We support buyers by offering relevant content in the form of recommendations and search results. For example, when a user returns to one of our marketplaces they are presented with a personalised homepage feed that immediately gives the user an overview of relevant ads that have recently been added or have not been viewed yet by the user. This brings the user straight back into the search experience. By clicking on one of the ads, the user is shown a detailed ad page with similar recommendations.
To understand the complexity of such a solution, it is important to understand the difference between online classifieds and your typical e-commerce business/platform. In an e-commerce business/platform you typically have a stock of several (perhaps hundreds) of items, whereas in online classifieds every item is unique and once sold it is removed from the marketplace. This means that a marketplace’s inventory is extremely volatile; as a result, traditional recommender algorithms do not perform very well in this context.
We also support sellers in adding new items to our marketplaces and making sure their ads perform well. Adding an item means the seller needs to provide pictures, a title, category, price, and a description of an item. We use computer vision to pre-fill some of these fields and to provide a price range that we believe the item will be sold at.
Getting these things right means that the item will be sold quickly, providing a good user experience for both seller and buyer and improving what is called the liquidity of the marketplace. We also provide sellers with a variety of stats about the performance of their ad and suggestions on how they might improve that performance.
Other areas where AI plays a role are more behind the scenes. For example, we have applications in advertising (targeting the right user for a given ad), marketing (engaging users with relevant content), and fraud detection (making sure fraudulent ads or ads that violate our policies don’t make it onto the platform).
AN: Do you think it helps to keep the flow of goods and services going in uncertain economic times?
TvK: Online classifieds are platforms that help people give their products a second life.
As well as getting some money in return for an item that’s no longer used by the seller, the sustainable nature of the transaction (reusing an item instead of buying a newly manufactured item) is an important motivator for many of our users.
Furthermore, our platforms reduce the socio-economic gap in society as certain expensive products (for example certain technology items) become available at a more affordable price.
AN: One problem that Adevinta highlighted in a blog post that is specific to recommender systems is the “cold-start problem” – can you explain what that is and how to overcome it?
TvK: The cold start problem in recommenders refers to items for which you have too little data (i.e. clicks) to apply your data-driven algorithm.
As described above, because our marketplaces have a highly volatile inventory compared to e-commerce, this problem is highly relevant. If we don’t have enough clicks to recommend an item, we fall back on natural language processing (NLP)-based techniques to determine which items are similar.
This requires a different data source (the metadata of an ad) and a different algorithmic approach in order to calculate similarity. Our personalisation services automatically determine if we have enough click data to build recommendations on or whether we have to fall back to the NLP-based approach.
AN: How do you ensure that the data you’re using for models is of sufficiently high quality?
TvK: All our marketplaces pipe their data to a centrally-created databus, which is basically a streaming solution that allows us to process behavioural data and other types of data in real-time.
We have recreated an in-house data quality monitoring platform that checks for basic anomalies in quality and allows for custom rules to check if quality levels are as expected. When we roll out a data product to one of our marketplaces, we check the data quality using this tooling and collaborate with the marketplaces team to improve the data quality if needed.
Once the product is live, a quality monitoring service monitors whether data quality drops below acceptable levels.
AN: Are you using a machine learning platform like SageMaker for your NLP models?
TvK: We have developed an internal machine learning (ML) platform based on Kubeflow and Kubernetes on which we run our services and workloads.
This ML platform allows us to have high utilisation of our computing clusters without having to pay an additional markup as we would for SageMaker. The ML platform furthermore allows us to integrate seamlessly with the rest of our CI/CD pipeline.
AWS SageMaker can still be useful for rapid prototyping, but we struggled to apply it successfully in a production setup due to the increased cost and the limited source control features.
AN: How important is the human element still in creative tasks? Do you foresee that changing much in the coming years?
TvK: Product development remains a highly creative and insightful task that many parties in our organisation are involved in.
Analytics helps us to make data-driven decisions, and toolkits and platforms help us to increase the time to market in rolling out a prototype, MVP, and finally the production-ready version, but the process of product development as a whole still requires very active human creativity.
AN: You recently presented a session at this year’s AI & Big Data Expo Europe, can you give us a brief overview for readers who were unable to attend?
In my talk at the AI & Big Data Expo, I explained some of the challenges that federated businesses face in building data products. I went into both the challenges and how we solved them in the areas of data collection, data processing, privacy, and experimentation. I then gave examples of data products we built in the personalisation and computer vision space.
You can view a recording of Tim van Kasteren’s session below:

Want to learn more about AI and big data from industry leaders? Check out AI & Big Data Expo taking place in Amsterdam, California, and London.
Explore other upcoming enterprise technology events and webinars powered by TechForge here.